МРТ-идентификация и классификация опухолей головного мозга с использованием DWT, PCA и машины опорных векторов ядра
DOI:
https://doi.org/10.47813/2782-2818-2024-4-1-0133-0152Ключевые слова:
Классификация, извлечение признаков, сегментация изображений, предварительная обработка, PNN, Дискретное вейвлет-преобразование, Вероятностная нейронная сетьАннотация
Классификация, сегментация и идентификация области инфекции на МРТ-изображениях опухолей головного мозга являются трудоемкими и итеративными процессами. Многочисленные анатомические структуры человеческого тела можно представить с помощью теории обработки изображений. С помощью базовых методов визуализации сложно увидеть аномальную структуру человеческого мозга. Неврологическую структуру человеческого мозга можно различить и уточнить с помощью метода магнитно-резонансной томографии. Подход МРТ использует ряд методов визуализации для оценки и регистрации внутренних особенностей человеческого мозга. В этом исследовании мы сосредоточились на стратегиях удаления шума, извлечении признаков из матрицы совместной встречаемости на уровне серого (GLCM) и сегментации областей опухоли головного мозга на основе дискретного вейвлет-преобразования (DWT) для минимизации сложности и повышения производительности. В свою очередь, это уменьшает любой шум, который мог остаться после сегментации из-за морфологической фильтрации. Сканирование МРТ головного мозга использовалось для проверки точности классификации и местоположения опухоли с использованием вероятностных классификаторов нейронных сетей. Точность классификатора и определение положения были проверены с помощью МРТ головного мозга. Эффективность предложенного подхода подтверждается экспериментальными результатами, которые показали, что нормальные и больные ткани можно отличить друг от друга на МРТ головного мозга с точностью около 100%.
Библиографические ссылки
Varuna Shree N., Kumar T. N. R. Identification and classification of brain tumor MRI images with feature extraction using DWT and probabilistic neural network. Brain Informatics. 2018; 5(1): 23-30. https://doi.org/10.1007/s40708-017-0075-5 DOI: https://doi.org/10.1007/s40708-017-0075-5
Sharma A., Singh J. Image denoising using spatial domain filters: A quantitative study. 2013 6th International Congress on Image and Signal Processing (CISP), Dec. 2013. https://doi.org/10.1109/cisp.2013.6744005 DOI: https://doi.org/10.1109/CISP.2013.6744005
Salem Ghahfarrokhi S., Khodadadi H. Human brain tumor diagnosis using the combination of the complexity measures and texture features through magnetic resonance image. Biomedical Signal Processing and Control, 2020; 61: 102025. https://doi.org/10.1016/j.bspc.2020.102025 DOI: https://doi.org/10.1016/j.bspc.2020.102025
Kwak N., Choi Chong-H. Input feature selection for classification problems. IEEE Transactions on Neural Networks. 2002; 13(1): 143-159. https://doi.org/10.1109/72.977291 DOI: https://doi.org/10.1109/72.977291
Amin J., Sharif M., Raza M., Saba T., Rehman A. Brain Tumor Classification: Feature Fusion. 2019 International Conference on Computer and Information Sciences (ICCIS), Sakaka, Saudi Arabia. 2019: 1-6. https://doi.org/10.1109/ICCISci.2019.8716449 DOI: https://doi.org/10.1109/ICCISci.2019.8716449
Ayadi W., Elhamzi W., Charfi I., Atri M. A hybrid feature extraction approach for brain MRI classification based on Bag-of-words. Biomedical Signal Processing and Control. 2019; 48: 144-152. https://doi.org/10.1016/j.bspc.2018.10.010 DOI: https://doi.org/10.1016/j.bspc.2018.10.010
Nagtode S. A., Potdukhe B. B., Morey P. Two dimensional discrete Wavelet transform and Probabilistic neural network used for brain tumor detection and classification. In 2016 Fifth International Conference on Eco-friendly Computing and Communication Systems (ICECCS). 2016. https://doi.org/10.1109/eco-friendly.2016.7893235 DOI: https://doi.org/10.1109/Eco-friendly.2016.7893235
Mathew A.R., Anto P.B. Tumor detection and classification of MRI brain image using wavelet transform and SVM. 2017 International Conference on Signal Processing and Communication (ICSPC). 2017: 75-78. https://doi.org/10.1109/CSPC.2017.8305810 DOI: https://doi.org/10.1109/CSPC.2017.8305810
Mao K. Z., Tan K.-C., Ser W. Probabilistic neural-network structure determination for pattern classification. IEEE Transactions on Neural Networks. 2000; 11(4): 1009-1016. https://doi.org/10.1109/72.857781 DOI: https://doi.org/10.1109/72.857781
Bahadure N.B., Ray A.K., Thethi H.P. Image Analysis for MRI Based Brain Tumor Detection and Feature Extraction Using Biologically Inspired BWT and SVM. International Journal of Biomedical Imaging. 2017; 2017: 1-12. https://doi.org/10.1155/2017/9749108 DOI: https://doi.org/10.1155/2017/9749108
Sabitha V., Nayak J., Reddy P. R. MRI brain tumor detection and classification using KPCA and KSVM. Materials Today: Proceedings. 2021. https://doi.org/10.1016/j.matpr.2021.01.714 DOI: https://doi.org/10.1016/j.matpr.2021.01.714
Rao C.S., Karunakara K. Efficient Detection and Classification of Brain Tumor using Kernel based SVM for MRI. Multimedia Tools and Applications. 2022. https://doi.org/10.1007/s11042-021-11821-z DOI: https://doi.org/10.1007/s11042-021-11821-z
Kumar K, Devi K.T. An Efficient Method for Brain Tumor Detection Using Texture Features and SVM Classifier in MR Images. Asian Pac J Cancer Prev. 2018; 19(10): 2789-2794. https://doi.org/10.22034/APJCP.2018.19.10.2789
Mandle A. K., Sahu S. P., Gupta G. Brain Tumor Segmentation and Classification in MRI using Clustering and Kernel-Based SVM. Biomedical and Pharmacology Journal. 2022; 15(2): 699-716. https://doi.org/10.13005/bpj/2409 DOI: https://doi.org/10.13005/bpj/2409
Jahidul I.M., Faruq O. Further Exploration of Deep Aggregation for Shadow Detection. Modern Innovations, Systems and Technologies. 2022; 2(3): 0312-0330. https://doi.org/10.47813/2782-2818-2022-2-3-0312-0330 DOI: https://doi.org/10.47813/2782-2818-2022-2-3-0312-0330
Naceur M. B., Saouli R., Akil M., Kachouri R. Fully Automatic Brain Tumor Segmentation using End-To-End Incremental Deep Neural Networks in MRI images. Computer Methods and Programs in Biomedicine. 2018; 166: 39-49. https://doi.org/10.1016/j.cmpb.2018.09.007 DOI: https://doi.org/10.1016/j.cmpb.2018.09.007
Lavanyadevi R., Machakowsalya M., Nivethitha J., Kumar A.N. Brain tumor classification and segmentation in MRI images using PNN. 2017 IEEE International Conference on Electrical, Instrumentation and Communication Engineering (ICEICE), Apr. 2017. https://doi.org/10.1109/iceice.2017.8191888 DOI: https://doi.org/10.1109/ICEICE.2017.8191888
Othman M.F., Basri M.A.M. Probabilistic Neural Network for Brain Tumor Classification. 2011 Second International Conference on Intelligent Systems, Modelling and Simulation. 2011: 136-138. https://doi.org/10.1109/ISMS.2011.32 DOI: https://doi.org/10.1109/ISMS.2011.32
Deepa B., Sumithra M. G., Kumar R. M., Suriya M. Weiner Filter based Hough Transform and Wavelet feature extraction with Neural Network for Classifying Brain Tumor. 2021 6th International Conference on Inventive Computation Technologies (ICICT), Jan. 2021. https://doi.org/10.1109/icict50816.2021.9358680 DOI: https://doi.org/10.1109/ICICT50816.2021.9358680
Thara S., Jasmine K. Brain tumour detection in MRI images using PNN and GRNN. 2016 International Conference on Wireless Communications, Signal Processing and Networking (WiSPNET), Mar. 2016. https://doi.org/10.1109/wispnet.2016.7566388 DOI: https://doi.org/10.1109/WiSPNET.2016.7566388
Zhang Y., Wang S., Wu L. A novel method for magnetic resonance brain image classification based on adaptive chaotic pso. Electromagn. Waves (Camb.). 2010; 109: 325-343. http://dx.doi.org/10.2528/PIER10090105 DOI: https://doi.org/10.2528/PIER10090105
Das S., Siddiqui N., Kriti N., Tamang S. P. Detection and area calculation of brain tumour from MRI images using MATLAB. International Journal of Computer Engineering In Research Trends. 2017; 4(1): 37-40. Available:
https://www.semanticscholar.org/paper/d7f3b185dcc8ed23f862b98a221988418e88c53f
Saraswathi D., Priya B. L., Punitha Lakshmi R. Brain tumor segmentation and classification using self-organizing map. In 2019 IEEE international conference on system, computation, automation and networking (ICSCAN). IEEE. 2019: 1-5. http://dx.doi.org/10.1109/ICSCAN.2019.8878763 DOI: https://doi.org/10.1109/ICSCAN.2019.8878763
Amin J., Sharif M., Raza M., Saba T., Anjum M. A. Brain tumor detection using statistical and machine learning method. Computer Methods and Programs in Biomedicine. 2019; 177: 69-79. https://doi.org/10.1016/j.cmpb.2019.05.015 DOI: https://doi.org/10.1016/j.cmpb.2019.05.015
Pandiselvi T., Maheswaran R. Efficient Framework for Identifying, Locating, Detecting and Classifying MRI Brain Tumor in MRI Images. Journal of Medical Systems. 2019; 43(7). https://doi.org/10.1007/s10916-019-1253-1 DOI: https://doi.org/10.1007/s10916-019-1253-1
Faruq O., Jahi I., Ahmed M. S., Hossain M. S. Brain tumor MRI identification and classification using DWT, PCA, and KSVM. Springer Research Square. 2023. https://doi.org/10.36227/techrxiv.21771329.v2 DOI: https://doi.org/10.21203/rs.3.rs-2562932/v1
Faruq O., Islam M. J., Ahmed M. S., Hossain M. S. Brain tumor MRI identification and classification using DWT, PCA, and KSVM. IEEE TechRxiv. 2023. https://doi.org/10.21203/rs.3.rs-2562932/v1 DOI: https://doi.org/10.36227/techrxiv.21771329.v2
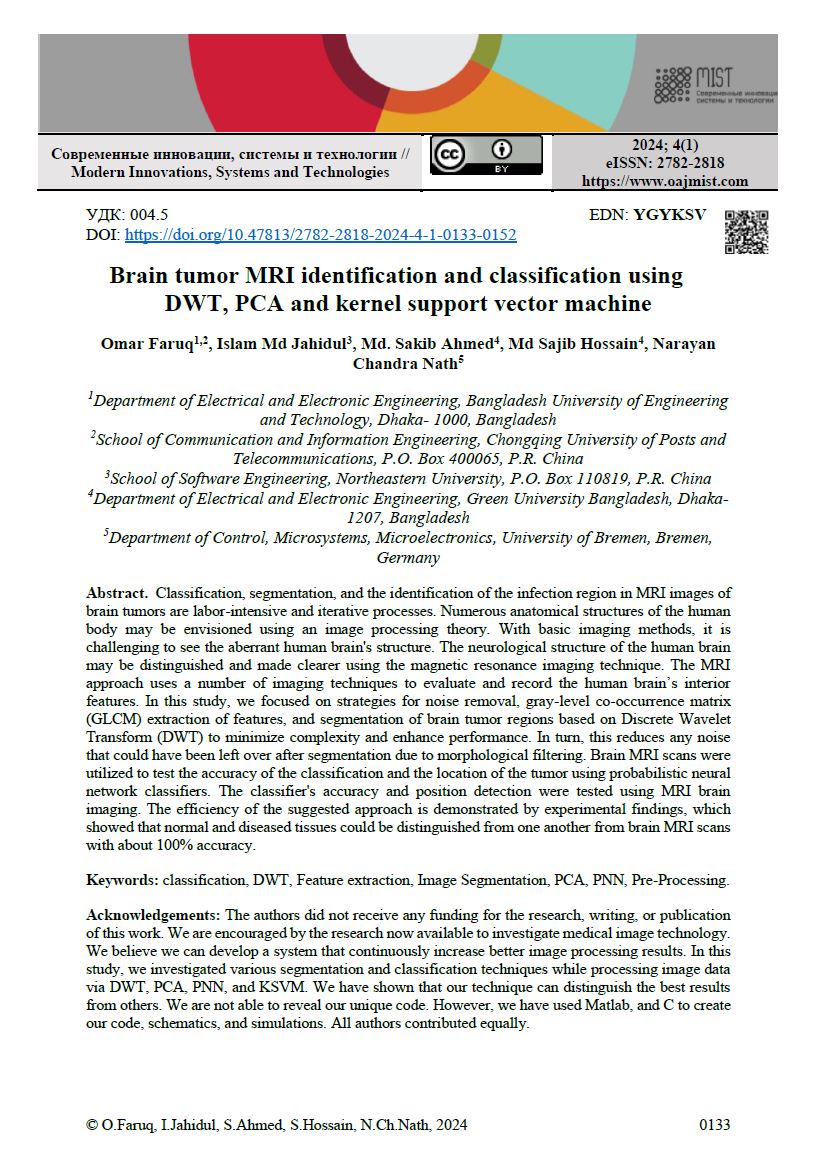
Загрузки
Опубликован
Как цитировать
Выпуск
Раздел
Лицензия
Copyright (c) 2024 O.Faruq, I.Jahidul, S.Ahmed, S.Hossain, N.Ch.Nath

Это произведение доступно по лицензии Creative Commons «Attribution» («Атрибуция») 4.0 Всемирная.
Журнал MIST - «Modern Innovations, Systems and Technologies» / «Современные инновации, системы и технологии» публикует материалы на условиях лицензии CreativeCommons Attribution 4.0 International (CC BY 4.0), размещенной на официальном сайте некоммерческой корпорации Creative Commons:
This work is licensed under a Creative Commons Attribution 4.0 International License.
Это означает, что пользователи могут копировать и распространять материалы на любом носителе и в любом формате, адаптировать и преобразовывать тексты, использовать контент для любых целей, в том числе коммерческих. При этом должны соблюдаться условия использования — указание автора оригинального произведения и источника: следует указывать выходные данные статей, предоставлять ссылку на источник, а также указывать, какие изменения были внесены.